There are many papers and abstracts and presentations that are available.
Real-world Limits to Algorithmic Intelligence (11 pages)
Recent theories of universal algorithmic intelligence, combined with the view that the world can be completely speci ed in mathematical terms, have led to claims about intelligence in any agent, including human beings. We discuss the validity of assumptions and claims made by theories of universally optimal intelligence in relation to their application in actual robots and intelligence tests. Our argument is based on an exposition of the requirements for knowledge of the world through observations. In particular, we will argue that the world can only be known through the application of rules to observations, and that beyond these rules no knowledge can be obtained about the origin of our observations. Furthermore, we expose a contradiction in the assumption that it is possible to fully formalize the world, as for example is done in digital physics, which can therefore not serve as the basis for any argument or proof about algorithmic intelligence that interacts with the world.
Complex Value Systems are Required to Realize Valuable Futures by Eliezer Yudkowsky
A common reaction to first encountering the problem statement of Friendly AI (“Ensure that the creation of a generally intelligent, self-improving, eventually superintelligent system realizes a positive outcome”) is to propose a single moral value which allegedly suffices; or to reject the problem by replying that “constraining” our creations is undesirable or unnecessary. This paper makes the case that a criterion for describing a “positive outcome”, despite the shortness of the English phrase, contains considerable complexity hidden from us by our own thought processes, which only search positive-value parts of the action space, and implicitly think as if code is interpreted by an anthropomorphic ghost-in-the-machine. Abandoning inheritance from human value (at least as a basis for renormalizing to reflective equilibria) will yield futures worthless even from the standpoint of AGI researchers who consider themselves to have cosmopolitan values not tied to the exact forms or desires of humanity.
Brain anatomy and artificial intelligence (35 pages)
The biological bases of mathematical competences: a challenge for AGI by Aaron Sloman
Aaron Sloman, from Britain, discussed “toddler theorems” – the symbolic understandings of the world that young children learn and create based on their sensorimotor and cognitive experiences. He challenged the researchers in the audience to understand and model the kind of learning and world-modeling that crows or human babies do, and sketched some concepts that he felt would be useful for this sort of modeling.
Extended Abstract
FACT:
Human children seem first to learn to talk using lots of learnt verbal patterns with which they get along quite well.Then they usually change, and apparently acquire a syntax-based competence that is far more powerful because it can generate entirely new utterances and enable them to understand novel utterances (in combination with compositional semantics).
But when that transition occurs, some of their learnt patterns are wrongly over-ridden by the new mechanism so they say “He hitted me”, “I runned home”, “She catched the ball”, whereas previously they would have said “He hit me” etc.
No amount of parental explanation, rebuking, repetition helps to correct the error, at that stage.
After a while, children spontaneously change and start coping with the exceptions alongside the grammatical rules. (e.g. “ran” not “runned”).
I assume that takes time because extending the newly constructed rule-based architecture to cope with exceptions is a non-trivial change (not easy even for a programmer to implement) whereas a purely pattern-based learning system doesn’t have rules that can have exceptions — there are just lots of learnt associations with different priorities.
CONJECTURE:
The first two parts of that process (learning re-usable patterns/associations, then replacing them with something more axiomatic and generative) occurs in many NON-linguistic competences in many species (humans, monkeys, cats, squirrels, crows, .
MIT’s Ed Boyden reviewed his recent work on optogenetics, one of the most exciting and rapidly developing technologies for imaging the brain – a very important area, given the point raised in the conference’s Special Track on Neuroscience and AGI, that the main factor holding back the design of AGI systems based on human brain emulation is currently the lack of appropriate tools for measuring what’s happening in the brain. We can’t yet measure the brain well enough to construct detailed dynamic brain simulations. Boyden’s work is one of the approaches that, step by step, is seeking to overcome this barrier.
Zhongzhi Shi, from the Chinese Academy of Sciences in Beijing, described his integrative AGI architecture, which incorporates aspects from multiple Western AGI designs into a novel overall framework. He also stressed the importance of cloud computing for enabling practical experimentation with complex AGI architectures like the one he described.
If you liked this article, please give it a quick review on ycombinator or StumbleUpon. Thanks
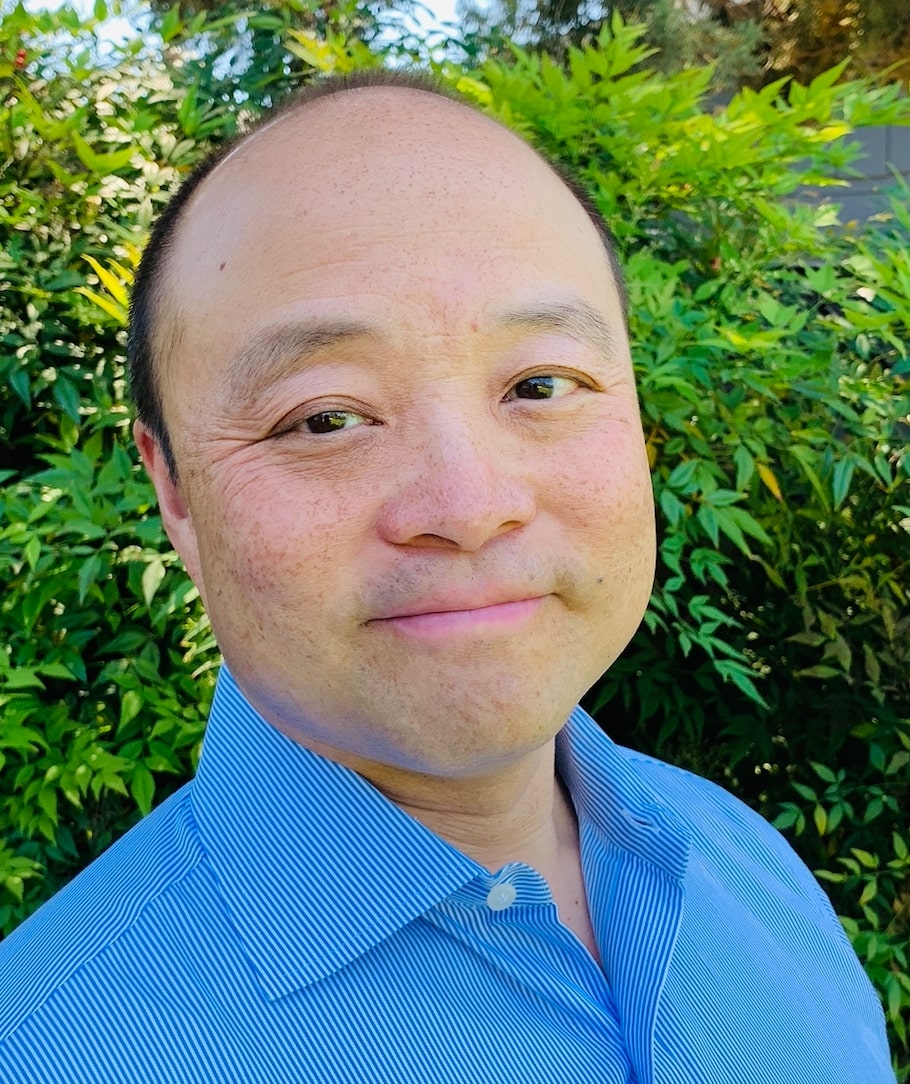
Brian Wang is a Futurist Thought Leader and a popular Science blogger with 1 million readers per month. His blog Nextbigfuture.com is ranked #1 Science News Blog. It covers many disruptive technology and trends including Space, Robotics, Artificial Intelligence, Medicine, Anti-aging Biotechnology, and Nanotechnology.
Known for identifying cutting edge technologies, he is currently a Co-Founder of a startup and fundraiser for high potential early-stage companies. He is the Head of Research for Allocations for deep technology investments and an Angel Investor at Space Angels.
A frequent speaker at corporations, he has been a TEDx speaker, a Singularity University speaker and guest at numerous interviews for radio and podcasts. He is open to public speaking and advising engagements.