Monica Anderson of Syntience
Why are so many things that are so easy for humans so difficult for computers ?
Computers use models
Science use models
people don’t
So AGI should not
Split things into reasoning and understanding
Reasoning -conscious, logic based deliberation
Understanding – subconscious intuition
Conscious reasoning has been overemphasized.
People receive 10 megabits/sec from the eyes and 1 megabits/sec from the skin and short change from elsewhere.
100 bits/second go the conscious layer.
reasoning is a paint thin layer on top of understanding
Introspection fallacy
Wishful thinking
I am a logical thinker
computers are logical
intelligence is logical
all Human Experience
most of experience is mundane (survival skills) (between rational and mystical – very small segments)
no miracles, no reductionist science
Intuitive understanding
Intuition is an algorithm
Invisibile to introspection
but not a mystical process
it is fallible (infallibilty is a selective memory)
Very difficult domains
1. chaotic systems that cannot be predicted long term
– deep complexity
-nonlinear responses
-state (memory)
2. Irreducible systems
-Open systems
-combinatorial explosion
-time variance
3. Amibiguous input data
4. Emergence
bizarre domains where models cannot be built
the world is bizarre
Life is bizarre
People are bizarre
language is bizarre
the mundane is bizarre
Technology holistic duplicates of scanned brains are not models
they will not provide the closed, compact
cannot restrict AGI implementation to the rational world
model free methods
Science creates models
Low level observation ==> Scientific intuition ==> Cross line to rational science with a scientific model
Observation ==> Engineer intuition ==> check preconditions and select a scientific model
model free methods subscientific intuition
low level observation ==>Experience & Intuition ==> Intuitive prediction
(gain from hanging out in pool halls)
Stronger to Weaker models
full scientific models
Partial models
Simulations
Statistics
Non-parametric models (weak model free method)
Pseudomodels (weak model free method)
Trial and Error (weak model free method)
Evolution is a strong model free method
model Free method zoo
Language – consultation, google
Evolution – genetic algorithms
Adaptation – PID controller / MFA controllers
Learning – Table lookup
Recognition – Repeat success
Discovery – Trial and Error
You are not guaranteed success using any of them.
No models given from outside
Gathering experience (learning is common
work everywhere – even in bizarre domains
require no intelligence to use
Disadvantages
– may not provide portable results
Artificial Intuition
* A very powerful model free method
* a fusion of other MFMs
* Provides understanding, learning, saliency, abstractions, semantics, and novelty
* 15000 lines of code
sensory input
recog
patterns
Emergent reductionism
Singularity and Skynet
Opinion and speculation
Intelligence/intuition is for prediction
the limits to prediction accuracy, precision, and time are not technological
limited by the bizarreness of the world
arrival of AGI may increase bizarreness
logic based machines have no advantage in the bizarre world
Logic based godlike infallible AGI is impossible
humans may already be almost as intuitive as anything can ever get
IMO at most we get machines just a little more intuitive than humans
A trillion that are not different
Enforced diversity
Create AGIs with enforced diversity by giving each one a unique education
An ecosystems of intelligences
intelligences may increase but slowly
no single agi to rule than all
RELATED SITES
syntience.com
artificial-intuition.com
monicasmind.com
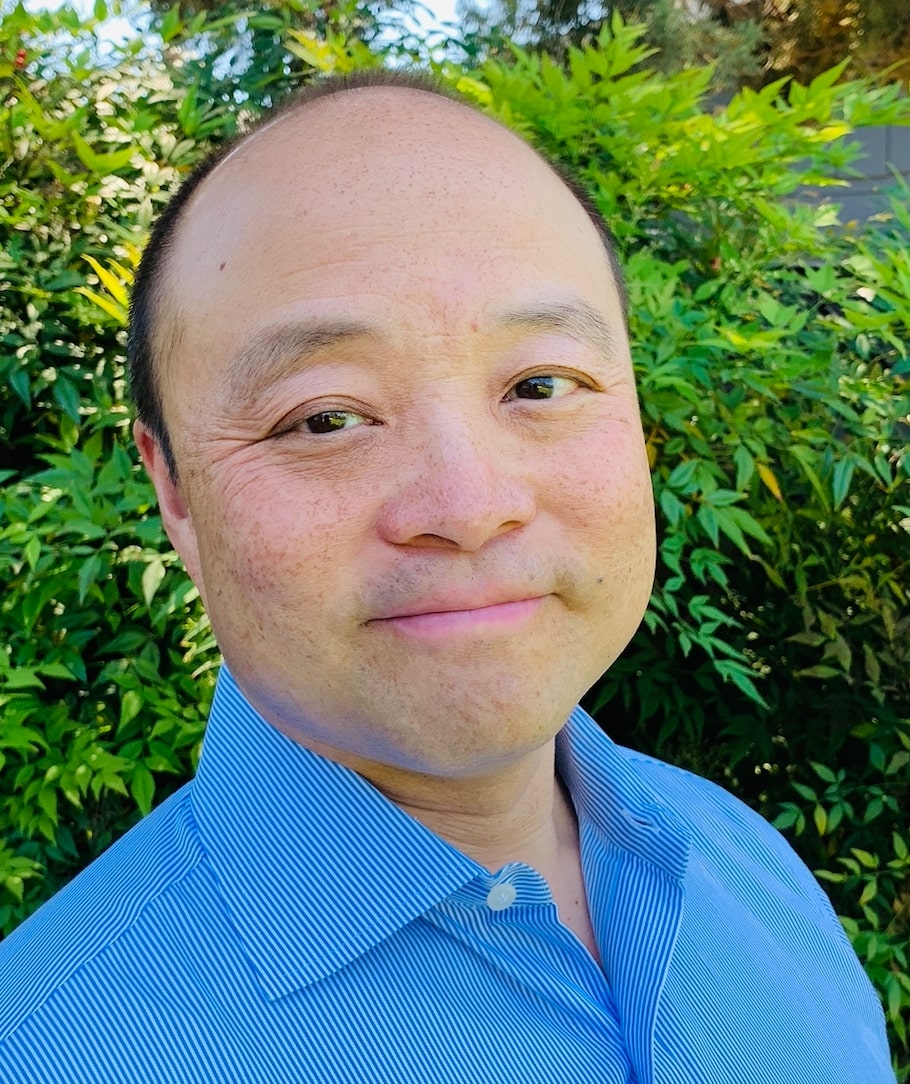
Brian Wang is a Futurist Thought Leader and a popular Science blogger with 1 million readers per month. His blog Nextbigfuture.com is ranked #1 Science News Blog. It covers many disruptive technology and trends including Space, Robotics, Artificial Intelligence, Medicine, Anti-aging Biotechnology, and Nanotechnology.
Known for identifying cutting edge technologies, he is currently a Co-Founder of a startup and fundraiser for high potential early-stage companies. He is the Head of Research for Allocations for deep technology investments and an Angel Investor at Space Angels.
A frequent speaker at corporations, he has been a TEDx speaker, a Singularity University speaker and guest at numerous interviews for radio and podcasts. He is open to public speaking and advising engagements.